In the mining industry, the pursuit of value optimization is a persistent and significant challenge. The strategic application of integrated simulation modelling across the entire value chain stands as a promising, yet largely underexploited, approach. Until now, its ability to drive substantial economic benefits has remained mostly unrealized. This is primarily due to the technical complexities involved, the requirement for in-depth expertise, and the need for complimentary, specialized tools. Informed by our recent experience with a large-scale integrated model that successfully unlocked hundreds of millions in value, this two-part series will delve into the various challenges, effective strategies, and the potential future impact of this innovative approach.
Decoding the Value Framework
The mining value framework serves as a key tool in categorizing and ranking the factors essential to a mine’s profitability and operational efficiency. This framework is instrumental in pinpointing where Discrete Event Simulation (DES) modelling can be most advantageous. Aligning modelling efforts with the knowledge derived from the framework allows for a strategic focus on elements that offer the greatest value for the business.
The figure below shows the value framework for a typical mine, noting that the relative performance of each element and its ranking vary greatly depending on the specific details of the mining operation, including location, type of commodity, and market conditions. Alongside each value driver is an assessment of relative influence discrete event simulation modelling can have on the driver: the length of the bar is the driver contribution to value with simulation influence shown as fill. The assessment of influence is based on deeper driver-tree analysis beyond the scope of this post and supported by nearly three decades of experience in deploying simulation models in the mining industry. It’s important to note that not all elements of the value framework are represented in the illustration. Specifically, aspects such as regulatory and community impacts, taxes and royalties, foreign exchange, and other factors that are not significantly influenced by simulation modelling have been intentionally omitted from this discussion. This omission is to maintain focus on areas where simulation modelling offers the most insight and impact, acknowledging that the full complexity of the value framework for a mining operation includes several other critical elements beyond the scope of this model-based analysis.
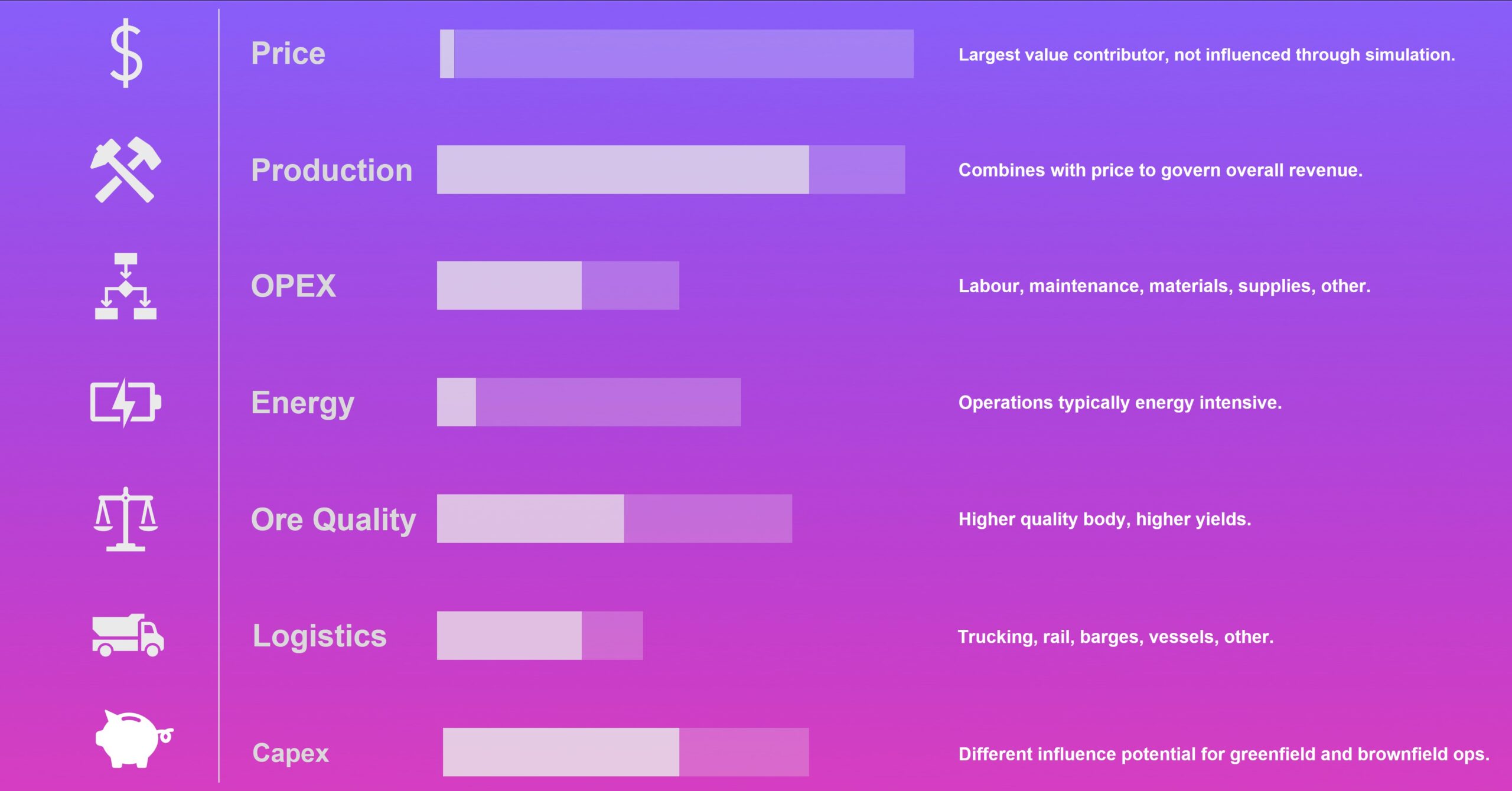
An examination of the framework’s elements, along with the influence of simulation on each, reveals production capacity as the contributor that provides the greatest impact in deriving value from simulation modelling. While there are benefits to be had from targeted simulation of other elements within the framework, and these should not be overlooked, it is the enhancement of production efficiency across the value chain that yields significant, transformative results. Therefore, the value framework indicates that the focus should be on enhancing incremental production at the lowest possible cost, identifying this as the key outcome goal. While this objective is clear in theory, its practical implementation presents a complex and demanding challenge, essential for unlocking the full potential of the mining value chain.
Deciphering the Challenges
Despite its considerable promise, the full realization of this technology’s potential in enhancing value remains largely untapped. McKinsey & Company (2020) stated that mine-to-market analysis can generate a 10 to 15 percent increase in earnings before interest, taxes, depreciation, and amortization (EBITDA) by optimizing throughput, product margins, and operating costs. However, in practical scenarios, these gains are rarely achieved. One key issue is that conventional approaches to simulation tend to conflate variability and uncertainty. While these analyses are typically thorough in exploring variability, they often fail to adequately address uncertainty, leading to a narrower analysis that misses a wider range of possible outcomes. To effectively navigate these challenges, it’s essential to widen the analysis aperture to fully encompass the uncertainties influencing the mining value chain. This leads us to confront two primary barriers impeding the extensive success and application of this approach: 1) scale and 2) the multifarious complexity inherent within the mining value chain.
Scale. Scale presents a technical challenge not because the mine value chain is expansive, but because it is comprised of many components, and sub-systems, that must be modelled to a high degree of fidelity to capture performance variance. The resultant model must then be subsequently powered with sufficient compute to provide the KPI precision necessary to effectively support value analysis. A moderately sized, well-designed model often requires several hours for a single replication, yielding substantial data that requires significant post computation resources to analyze. Optimization of a simple objective function on simulation models of this scale is not feasible using reinforcement learning techniques and therefore requires a suite of methodologies (e.g. application of modified Theory of Constraints and associated regression analysis) and bespoke supplemental tools (deterministic production calculators and stochastic production simulators).
Multifarious complexity. Several characteristics unique to the mine value chain present another set of challenges for integrated modelling.
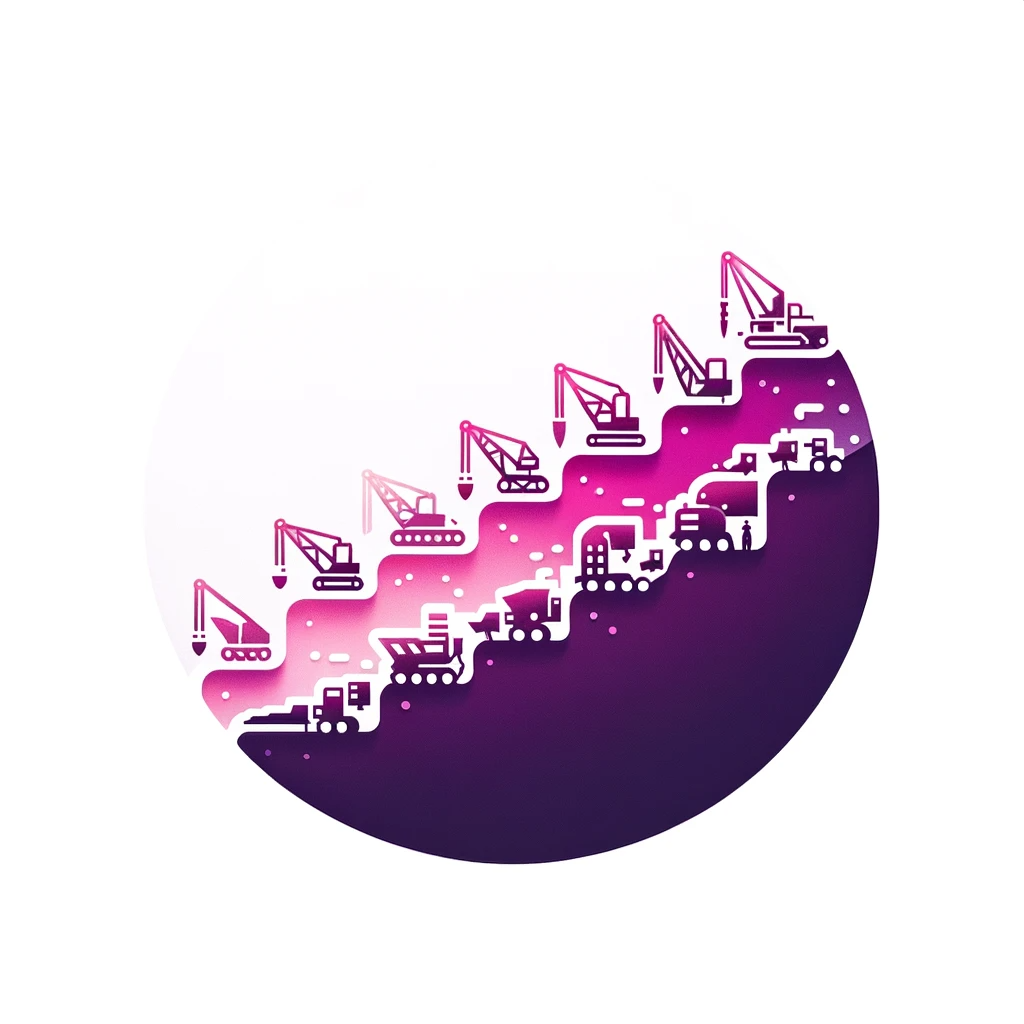
Spatial evolution. In all mining methods, the process of extracting ore from the orebody creates an underlying system that is in a constant state of change as new areas are developed, and old ones are depleted or abandoned. This spatial evolution creates a non-stationary, dynamic mine boundary, one in which changing geological features and evolving extraction techniques make it difficult to apply standard modelling techniques that typically assume a static or slowly changing environment.
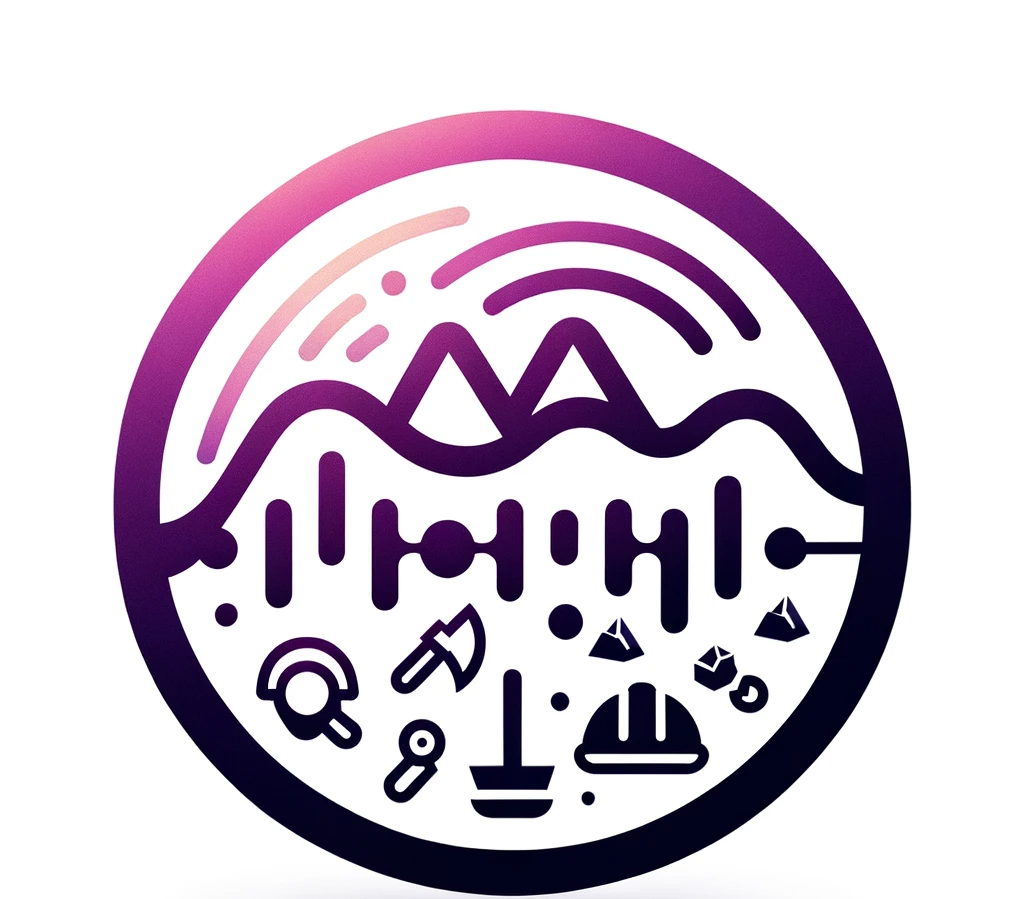
High variability. The mine value chain encompasses operations that span key areas such as mining, hoisting, processing, and outbound logistics. Each area features a complex network of numerous unit operations, often in the hundreds. These operations are characterized by distinct features, influenced by equipment, human factors, and design. A significant challenge for integrated mine modelling is the inherent variability across these operations. This variability is not merely a product of individual unit performance; it’s compounded by the intricate interdependencies among these units, often including feedback considerations. Changes in one operation can precipitate unanticipated effects in others, exacerbating the effect of variability and creating wide performance outcomes throughout the chain.
The challenge of modelling mining operations is further complicated by the temporal nature of variability, which manifests differently over short, medium, and long-term periods. A concern in this context is fidelity, particularly the lack of ability in many models to capture variability between ‘events’. Short term variation is present in several forms throughout the value chain. Operating schedules, critical mining sequences, and small storage buffers, among many other elements, often have performance variation that changes rapidly. To accurately represent their short-term variations and intricate interactions within the broader mining operation, high-fidelity modelling is required. This necessitates a hybrid event-to-event plus continual modelling approach, which effectively captures these subtleties. Advanced simulation methods are integral to this process, enabling the creation of models that thoroughly track variabilities, leading to a richer understanding of mining operations. Such methods overcome traditional simulation limitations, preventing operational misunderstandings.
This complexity, coupled with the dynamic and temporally nuanced nature of variability in the mining value chain, underscores the challenges in accurately capturing its full impact and predicting potential performance outcomes over various time scales.
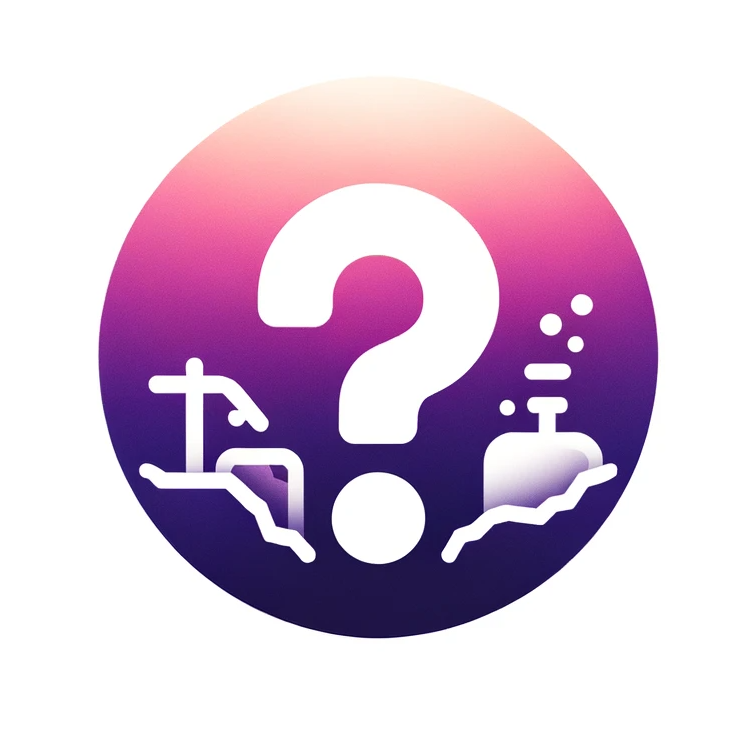
High uncertainty. Uncertainty is a pervasive challenge in the mining industry, stemming from various sources. Geological factors such as ore body characteristics, market conditions like price volatility and demand fluctuations, and operational aspects including equipment reliability and system performance all contribute to this uncertainty. Additionally, and often overlooked, are the uncertainties linked to capital allocation decisions. These involve predicting the performance enhancement or returns from investments in new technologies, infrastructure, or operational changes, and are crucial for unlocking value. A key challenge arises in the application of integrated simulation modeling. While the Monte Carlo nature of DES is by nature proficient at managing operational variability, it falls short in accurately capturing the broader spectrum of outcome uncertainties. This results in an underrepresentation of the impact of uncertainty, diminishing the effectiveness of simulations that rely solely on DES for quantitative performance outcome and analysis. The range of outcomes due to uncertainty typically exceeds those attributable to variability, highlighting a significant gap. This disparity underscores the need for enhanced methodologies or tools that can more effectively integrate and analyze the impact of uncertainty, ensuring a more comprehensive approach to addressing these challenges.
Combined, these complexities limit the effectiveness of conventional DES in fully capturing the range of outcomes in capital deployment analysis. To address these limitations, the adoption of additional tools and methodologies is crucial. They offer a more comprehensive view of the value chain and enable a more thorough evaluation of strategies, by locating bottlenecks, validating potential mitigations, and truly optimizing capital allocation. These insights pave the way for the next installment of our series, which will delve deeper into the integrated simulation approach and its role in redefining value optimization in the mining sector.